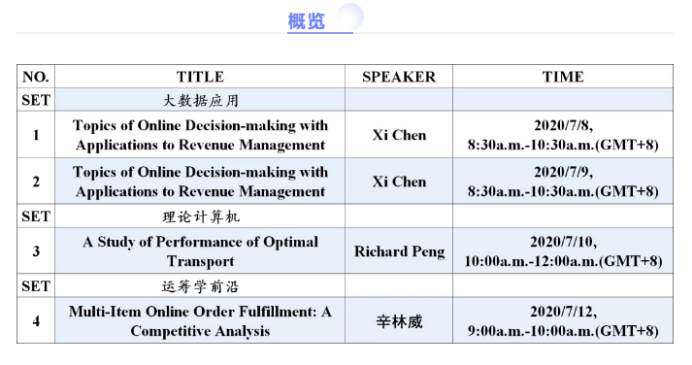
1
时间TIME
2020/7/8, 8:30a.m.-10:30a.m.(GMT+8)
会议室Zoom Rooms
Zoom会议ID: 63205123577
参会链接: https://zoom.com.cn/
j/63205123577
密码: 123456
主讲人SPEAKER
Xi Chen is an associate professor at Stern School of Business at New York University. Before that, he was a Postdoc in the group of Prof. Michael Jordan at UC Berkeley. He obtained his Ph.D. from the Machine Learning Department at Carnegie Mellon University (CMU); and his Masters degree in Industry Administration and Operations Research from the Tepper School of Business at CMU.
He studies high-dimensional statistics, online machine learning, large-scale stochastic optimization and applications to revenue management. He received NSF CAREER Award, Simons-Berkeley Research Fellowship, faculty research award from a wide range of technology companies, such as Google, Facebook, Adobe, J.P. Morgan, Bloomberg, and Alibaba. Moreover, he was listed on Forbes 30 under 30.
系列SET
大数据应用
题目TITLE
Topics of Online Decision-making with Applications to Revenue Management
(讲座语言:中文)
摘要ABSTRACT
This mini-course covers the foundations of online learning based on the multi-armed bandit framework and applications to revenue management.
The course contains two lectures. Each lecture is about 2 hours. The first lecture provides the foundations of online learning. We will discuss topics such as epsilon-greedy algorithm, UCB, Thompson sampling algorithm and applications to dynamic pricing. The second lecture discusses several of my recent research on assortment optimization for online recommendation. The course is suitable for senior undergraduates or graduate students.
2
时间TIME
2020/7/9, 8:30a.m.-10:30a.m.(GMT+8)
会议室Zoom Rooms
Zoom会议ID: 67471897341
参会链接: https://zoom.com.cn/
j/67471897341
密码: 123456
主讲人SPEAKER
Xi Chen is an associate professor at Stern School of Business at New York University. Before that, he was a Postdoc in the group of Prof. Michael Jordan at UC Berkeley. He obtained his Ph.D. from the Machine Learning Department at Carnegie Mellon University (CMU); and his Masters degree in Industry Administration and Operations Research from the Tepper School of Business at CMU.
He studies high-dimensional statistics, online machine learning, large-scale stochastic optimization and applications to revenue management. He received NSF CAREER Award, Simons-Berkeley Research Fellowship, faculty research award from a wide range of technology companies, such as Google, Facebook, Adobe, J.P. Morgan, Bloomberg, and Alibaba. Moreover, he was listed on Forbes 30 under 30.
系列SET
大数据应用
题目TITLE
Topics of Online Decision-making with Applications to Revenue Management
(讲座语言:中文)
摘要ABSTRACT
This mini-course covers the foundations of online learning based on the multi-armed bandit framework and applications to revenue management.
The course contains two lectures. Each lecture is about 3 hours. The first lecture provides the foundations of online learning. We will discuss topics such as epsilon-greedy algorithm, UCB, Thompson sampling algorithm and applications to dynamic pricing. The second lecture discusses several of my recent research on assortment optimization for online recommendation. The course is suitable for senior undergraduates or graduate students.
3
时间TIME
2020/7/10, 10:00a.m.-12:00a.m.(GMT+8)
会议室Zoom Rooms
Zoom会议ID:61330583950
参会链接:https://zoom.com.cn/j/61330583950
密码:123456
主讲人SPEAKER
Richard Peng is an assistant professor in the School of Computer Science at the Georgia Institute of Technology. His research interests are in the design, analysis, and implementation of efficient algorithms and data structures, with a focus on graphs and sparse matrices. Richard received his BMath from Waterloo, his PhD from CMU, and was a postdoc at MIT. He has received the NSF Career Award, the Microsoft Research PhD Fellowship, and the CMU SCS Distinguished Dissertation Award.
系列SET
理论计算机
题目TITLE
A Study of Performance of Optimal Transport
摘要ABSTRACT
This talk will discuss recent progresses on computing optimal transport (OT) distances, which are equivalent to node-capacitated min-cost max-flows in bipartite graphs. OT, or Wasserstein, distances between probability distributions have direct applications to learning and clustering, and have recently gained momentum in natural language processing and image retrieval.
The first half of this talk will survey various methods for computing OT, including combinatorial graph algorithms such as network simplex and auction, as well as the widely used Sinkhorn iteration. We then present a new combinatorial algorithm that improves upon the classical Kuhn-Munkres algorithm in the low accuracy regime by leveraging sparsity of the admissible arcs.
The second half of the talk will revolve around theoretical analogs of such sparsity based speedups. We will describe an algorithm that computes exact optimal transport on graphs with n vertices and integer edge weights between [1,W] in randomized O(n^{2 + o(1)} logW) time. This also gives the first runtime improvement for dense bipartite matching in over 30 years. This algorithm is based on combining second-order/interior point methods with dynamic graph sparsification data structures.
4
时间TIME
2020/7/12, 9:00a.m.-10:00a.m.(GMT+8)
会议室Zoom Rooms
Zoom会议ID:913 3479 7174
参会链接:https://chicagobooth.zoom.us/j/91334797174?
pwd=TUJBSTZMOUtXWjN5RHlOZ282Rmswdz09
会议密码:273102
主讲人SPEAKER
辛林威博士是美国芝加哥大学Booth商学院助理教授(运营管理方向)。主要研究方向包括:库存管理,供应链管理,收益管理,不确定性优化,数据驱动的智能决策。他的学术研究获得过的荣誉包括:INFORMS应用概率学会颁发的最佳论文奖(2019),INFORMS George E. Nicholson最佳学生论文奖(2015),INFORMS最佳青年学者论文奖第二名(2015),INFORMS生产与服务运作管理最佳学生论文入围奖(2014),华人学者管理科学与工程协会颁发的最佳论文奖(2017)。他的研究成果发表在《Operations Research》,《Management Science》等学术期刊上。他曾经就职于沃尔玛全球电子商务研究中心及IBM Watson研究中心,与业界的研究合作包括:沃尔玛全球电子商务,阿里巴巴集团。
系列SET
运筹学前沿
题目TITLE
Multi-Item Online Order Fulfillment: A Competitive Analysis
(讲座语言:中文)
摘要ABSTRACT
The boom of e-commerce in the globe in recent years has expedited the expansion of fulfillment infrastructures by e-retailers. While e-retailers are building more warehouses to offer faster delivery service than ever, the associated fulfillment costs have skyrocketed over the past decade. In this paper, we study the problem of minimizing fulfillment costs, in which the e-retailer must decide which warehouse(s) to fulfill each order from, subject to warehouses’ inventory constraints. The e-retailer can split an order, at an additional cost, and fulfill it from different warehouses.
We focus on a two-layer distribution network that has been implemented in practice by major e-retailers. We analyze the performance of fulfillment policies that do not rely on demand forecasts. We show that, under an assumption that aligns with the practice of most e-retailers, a myopic policy achieves a constant performance ratio compared to an optimal clairvoyant algorithm. We further show that this ratio is tight. More generally, we characterize the performance guarantees of the myopic policy in terms of the cost parameters of the model. Finally, we complement our theoretical results by conducting a numerical study and demonstrate the good performance of the myopic policy.
This is joint with Yanyang Zhao (Chicago Booth) and Xinshang Wang (Alibaba).